We consider a classical task of signal denoising:
create an estimate u of an original signal
z from its noisy measurement f, where
f = z + n
and n denotes an additive noise function.
Various methods have been proposed to remove the noise from z
without sacrificing important structures such as edges, including
rank-order filtering, mathematical morphology,
stochastic methods,
adaptive smoothing,
wavelet techniques,
partial differential equations (PDEs)
and variational methods.
Although these classes of methods serve the same purpose, relatively few
publications examine their similarities and differences, in order to
transfer results from one of these classes to the others, or to design
hybrid methods that combine the advantages of different classes.
By studying their relations, we have tried to fill this gap and
contribute to a better understanding of the methods involved.
-
Relations between regularization functionals and nonlinear diffusion
Both nonlinear diffusion and regularization functionals are popular methods
for image denoising. We found out that both techniques are closely related,
as the Euler-Lagrange equations used for minimizing the energy functional
is a partial differential equation, very similar to the diffusion equation
used in nonlinear diffusion [1]. For the special case
of total variation (TV) regularization and TV diffusion, we were even able
to show that in 1D both techniques yield equivalent results
[4,6].
-
Relations between wavelet shrinkage and nonlinear diffusion
We also studied correspondences between discrete Haar wavelet shrinkage
and nonlinear diffusion. Starting with the very simple case of only two pixels,
we found out that soft haar wavelet shrinkage and TV diffusion are equal
[2]. It turned out that this equivalence is not restricted
to the two-pixel case. Also in the general 1D case, the methods can be made
equivalent by choosing appropriate diffusivity or shrinkage functions
[5,8]. The connections allowed us
to design hybrid methods which combine the speed of wavelet shrinkage and the
robustness of iterative methods [3]. For the
two-dimensional situation, the diffusion inspired us to design Haar
wavelet filters with improved rotation invariance [7].
-
Equivalence of soft wavelet shrinkage, TV regularization, TV diffusion, and SIDEs
Putting both results together, three popular denoising techniques could
be shown to be completely equivalent in the discrete 1D setting, when choosing
a special shrinkage function, potential, and diffusivity. Furthermore, also the
dynamical system of SIDEs, which is based on merging operations, is in this case
equivalent to the other three methods [6].
[1] |
O. Scherzer, J. Weickert:
Relations between regularization and diffusion filtering.
Journal of Mathematical Imaging and Vision, Vol. 12, 43-63, 2000.
Revised version of
Technical Report DIKU-98/23,
Dept. of Computer Science,
University of Copenhagen, Denmark, 1998.
|
[2] |
G. Steidl, J. Weickert:
Relations between soft wavelet shrinkage and total
variation denoising.
In L. Van Gool (Ed.): Pattern Recognition.
Lecture Notes in Computer Science, Vol. 2449, Springer, Berlin,
198-205, 2002.
|
[3] |
P. Mrázek, J. Weickert, G. Steidl and M. Welk
On iterations and scales of nonlinear filters
O. Drbohlav (ed.): Computer Vision Winter Workshop 2003,
Valtice, Czech Republic, pp.61-66. Czech Pattern Recognition Society, 2003.
|
[4] |
T. Brox, M. Welk, G. Steidl, J. Weickert:
Equivalence results for TV diffusion and TV regularisation.
L. D. Griffin, M. Lillholm (Eds.): Scale Space Methods in Computer
Vision.
Lecture Notes in Computer Science, Vol. 2695, Springer, Berlin, 86-100,
2003.
|
[5] |
P. Mrázek, J. Weickert and G. Steidl
Correspondences between wavelet shrinkage and nonlinear diffusion
L.D. Griffin and M. Lillholm (Eds.):
Scale-Space 2003, LNCS 2695, pp. 101-116. Springer, 2003.
|
[6] |
G. Steidl, J. Weickert, T. Brox, P. Mrázek and M. Welk:
On the equivalence of soft wavelet shrinkage, total variation diffusion,
total variation regularization, and SIDEs.
SIAM Journal on Numerical Analysis, Vol. 42, No. 2, 686-713, 2004.
An extended version appeared as
Technical Report No. 94, Department of Mathematics,
Saarland University,
Saarbrücken, Germany, August 2003.
|
[7] |
P. Mrázek and J. Weickert
Rotationally invariant wavelet shrinkage
B. Michaelis and G. Krell (Eds.):
DAGM 2003, LNCS 2781, pp. 156-163. Springer, 2003.
|
[8] |
P. Mrázek, J. Weickert and G. Steidl
Diffusion-inspired shrinkage functions
and stability results for wavelet denoising
Preprint no. 96, Department of Mathematics,
Saarland University, Saarbrücken, Germany, 2003.
|
Image smoothing example
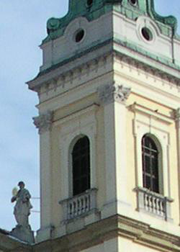 |
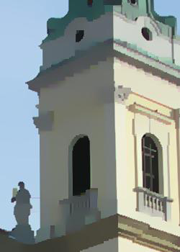 |
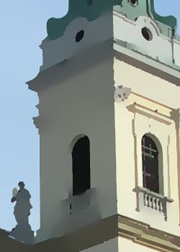 |
 |
 |
 |
Input image and its detail. |
Result of classical shift-invariant
Haar wavelet shrinkage:
typical blocky artefacts and misaligned colour channels
due to independent processing of the RGB channels. |
Shift-invariant Haar wavelet filtering with
diffusion-inspired shrinkage rules
improves rotation invariance [7]
and avoids colour artefacts.
|
|